The Essential Guide to Data Labeling in Machine Learning
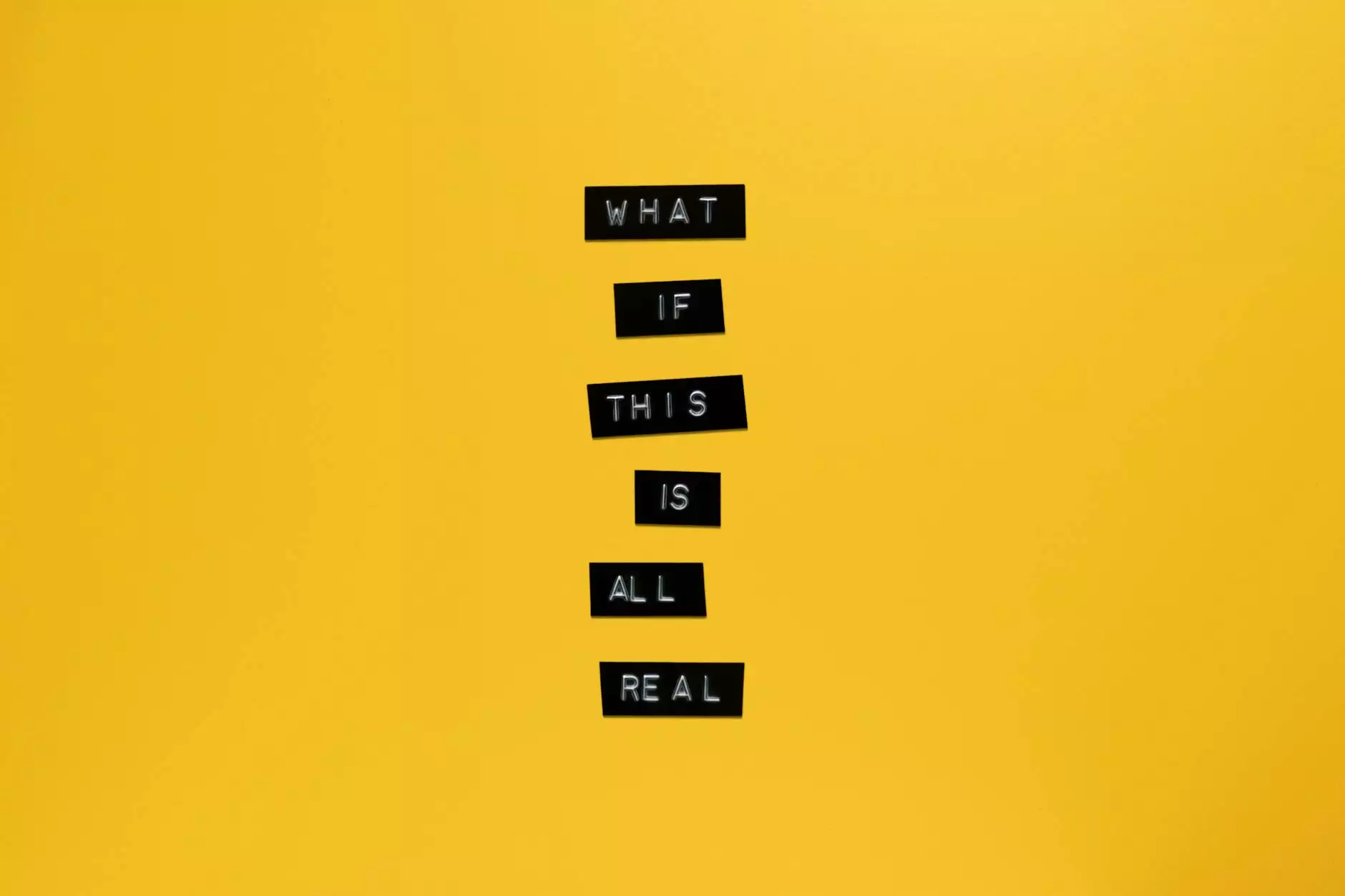
Data labeling is one of the cornerstones of machine learning. In a world where artificial intelligence (AI) is rapidly transforming industries, understanding the significance of proper data annotation becomes crucial. This comprehensive article delves into the intricacies of data labeling, its vital role in machine learning, and how utilizing a streamlined data annotation platform, such as KeyLabs.ai, can enhance the efficacy and accuracy of your machine learning models.
What is Data Labeling?
Data labeling is the process of tagging or annotating raw data to make it understandable for machine learning systems. These labels can take various forms, such as text labels, bounding boxes, polygons, or even audio transcriptions, depending on the data type. The primary objective of data labeling is to provide high-quality training data to machine learning algorithms, allowing them to learn patterns and make predictions.
Why is Data Labeling Critical in Machine Learning?
The effectiveness of any machine learning model hinges significantly on the quality and quantity of data it is trained on. Here’s why data labeling is essential:
- Quality Training Data: Well-labeled data ensures that the model learns from accurate information, reducing errors in predictions.
- Model Performance: Quality data labeling directly influences the performance and reliability of the AI models. Poorly labeled data can lead to misclassification, causing models to fail in real-world applications.
- Scalability: As data continues to grow, efficient labeling processes become necessary for scalable machine learning solutions.
- Facilitates Supervised Learning: Most machine learning algorithms require labeled data for supervised learning. This method makes it easier for the model to learn from a known dataset and apply that learning to new, unseen data.
Types of Data Labeling Techniques
Data labeling can be divided into several categories based on the data type and the annotation method used:
- Image Annotation: Involves labeling images with bounding boxes, polygons, or image segmentation. Commonly used for training computer vision models.
- Text Annotation: This includes labeling parts of text, entity recognition, and sentiment analysis, essential for natural language processing (NLP) tasks.
- Audio Annotation: Labels audio clips to help machines understand spoken words, emotions, and sounds. Critical for speech recognition systems.
- Video Annotation: Involves marking various objects, actions, and behaviors within videos. This is important for applications like security systems and autonomous driving.
Challenges in Data Labeling
While data labeling is essential, it is not without its challenges:
- Volume of Data: With the exponential growth of data, the sheer volume makes it challenging to label everything effectively.
- Consistency: Achieving consistency in labeling across different annotators is difficult, yet vital for model accuracy.
- Cost: The process of data labeling can be labor-intensive and costly, particularly for large datasets.
- Human Error: Mistakes made during manual labeling can lead to poor quality data, adversely affecting model performance.
Solutions for Efficient Data Labeling
To overcome these challenges, businesses are increasingly turning to advanced data annotation tools available on platforms like KeyLabs.ai. Here's how they can help:
- Automated Annotation: Some tools utilize AI and machine learning for automated image and text labeling, significantly reducing the workload.
- Quality Control Mechanisms: Implementing review processes and using multiple annotators can ensure consistency and accuracy in labels.
- Scalable Solutions: Cloud-based platforms allow for scaling operations based on demand, accommodating large volumes of data effortlessly.
- Cost-Effective Solutions: Outsourcing data annotation or using in-house teams trained with the right tools can optimize costs and improve turnaround times.
KeyLabs.ai: Your Partner in Data Annotation
When it comes to data labeling in machine learning, KeyLabs.ai stands out as a premier data annotation platform. With a commitment to quality and efficiency, KeyLabs.ai offers a host of tools designed to enhance the labeling process giving your AI projects the precision they need.
Features of KeyLabs.ai
KeyLabs.ai provides an array of features tailored to meet the needs of businesses:
- User-Friendly Interface: Designed for simplicity, enabling teams to label data without extensive training.
- Customizable Workflows: Flexibility to set up annotation projects that suit specific requirements and timelines.
- Expert Annotators: Access to trained professionals who understand the nuances of various data types and industries.
- Real-Time Collaboration: Facilitates seamless communication and updates between team members, ensuring that everyone stays aligned.
The Future of Data Labeling in Machine Learning
As machine learning continues to evolve, the importance of high-quality data labeling becomes even more pronounced. Here’s what the future holds:
- Increased Use of AI for Automation: The integration of AI in the labeling process will enhance speed and reduce human errors.
- Collaborative Platforms: More companies will implement platforms that allow for collaboration across teams, driving more efficient data processes.
- Higher Demand for Speed and Quality: The need for rapid, accurate annotations will necessitate advancements in labeling technologies.
- Focus on Specialized Labeling: Emerging fields will require more specialized labelers for tasks like medical imaging and autonomous vehicle training.
Conclusion
In the realm of machine learning, data labeling is not just an incidental step; it is a foundational element that influences the success of AI applications. The insights shared in this article aim to highlight the criticality of effective data annotation and how platforms like KeyLabs.ai can be indispensable allies in achieving your data objectives. By embracing high-quality data labeling practices, businesses can unlock the true potential of their machine learning efforts, driving innovation and competitive advantage in their respective fields.
For more information on how to transform your machine learning projects with top-tier data annotation tools, visit KeyLabs.ai.
data labeling machine learning