The Power of Agriculture Datasets for Machine Learning
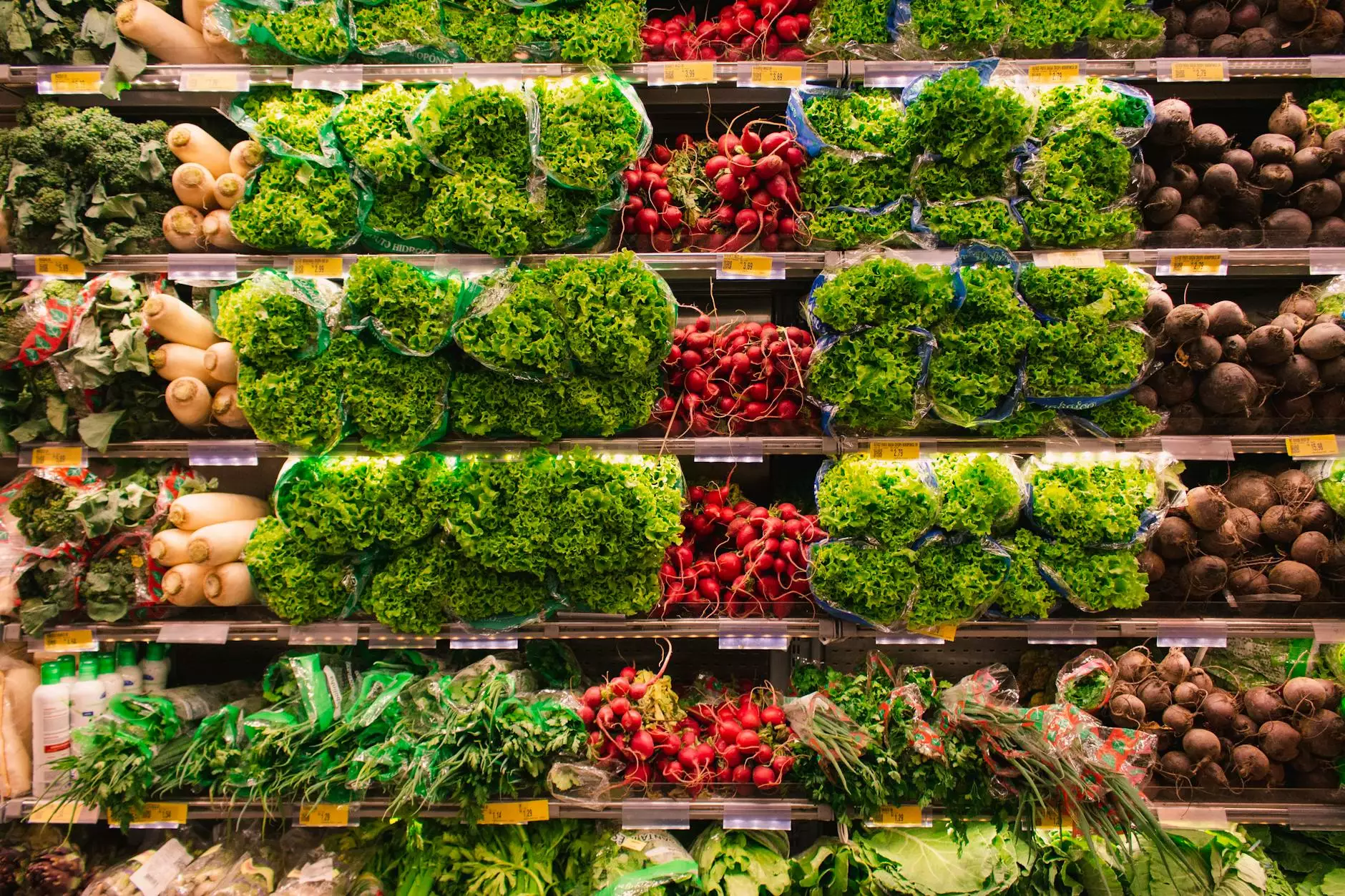
Machine learning has transformed various industries, and one of the most impactful sectors it influences is agriculture. With the rise of technology in farming, leveraging an agriculture dataset for machine learning has become a game-changer. This article delves into how such datasets can optimize agricultural practices, enable predictive analytics, and contribute to sustainable farming.
Understanding Agriculture Datasets
An agriculture dataset consists of structured and unstructured data that relates to agricultural practices. This could include anything from crop yields, soil health, weather conditions, pest occurrences, and more. The key to unlocking the benefits of machine learning lies within these datasets. Here are some essential types of agriculture datasets:
- Crop Yield Data: Historical data on crop production, which can be used to predict future yields based on various factors.
- Soil Composition Data: Information on soil types, nutrient levels, and pH, essential for determining which crops can thrive in certain areas.
- Weather Data: Daily or seasonal weather patterns, temperature fluctuations, and precipitation levels, which affect agricultural output.
- Pest and Disease Data: Records of pest populations and crop diseases that can threaten agricultural productivity.
- Market Data: Price trends, demand fluctuations, and supply chain information that help farmers make informed decisions about planting and selling.
The Role of Machine Learning in Agriculture
Machine learning algorithms can analyze these vast datasets to identify patterns and provide insights that are not easily detectable by humans. Here are some key roles that machine learning plays in agriculture:
1. Precision Farming
This technique uses data analytics to optimize field-level management with regards to crop farming. Machine learning models can determine optimal planting times, fertilizer usage, and irrigation schedules to enhance productivity while minimizing waste and environmental impact.
2. Crop Disease Prediction
By analyzing historical data on crop diseases along with current environmental data, machine learning can predict the likelihood of disease outbreaks. Farmers can use this information to implement preventive measures, enhancing crop health and yield.
3. Yield Prediction
Machine learning models can forecast crop yields by analyzing various factors, including historical yield data, current weather conditions, and soil health. This predictive capability allows farmers to make better planting and marketing decisions.
4. Automated Irrigation Systems
Machine learning can optimize irrigation practices by analyzing soil moisture levels and weather patterns. These smart systems can automate watering schedules, ensuring crops receive the right amount of water at the right time.
Benefits of Using Agriculture Datasets for Machine Learning
Utilizing agriculture datasets for machine learning comes with numerous benefits:
1. Increased Efficiency
By leveraging data-driven insights, farmers can enhance their operational efficiency. Machine learning helps identify the best practices to maximize yield while preserving resources.
2. Sustainability
Machine learning can contribute significantly to sustainable farming practices. By minimizing the use of water, pesticides, and fertilizers through informed decision-making, farmers can reduce their environmental impact.
3. Enhanced Decision-Making
Farmers equipped with predictive analytics are better prepared to make decisions that affect their operations, from choosing crop types to timing their harvests.
4. Risk Management
Machine learning models can predict adverse weather conditions or pest outbreaks, allowing farmers to take proactive measures. This foresight helps mitigate risks in production and finance.
Challenges in Using Agriculture Datasets
While the potential benefits are vast, several challenges exist when working with agriculture datasets:
- Data Quality: The effectiveness of machine learning models heavily depends on the quality of the data. Inaccurate or incomplete data can lead to erroneous insights.
- Data Integration: Combining different data sources - such as weather, soil, and market data - can be a complex task, requiring robust data management solutions.
- Privacy and Security: Sensitive information about farming practices and land use may raise privacy concerns. Ensuring data security is paramount.
- Technological Barriers: Not all farmers have access to advanced technological tools needed to implement machine learning solutions effectively.
Getting Started with Agriculture Datasets for Machine Learning
For those interested in harnessing the power of agriculture datasets for machine learning, here’s how to get started:
1. Identify Relevant Datasets
Start by sourcing relevant datasets. Public repositories, governmental databases, and agricultural research organizations often provide valuable data. Websites like Kaggle, UCI Machine Learning Repository, and others can be great resources.
2. Data Preprocessing
Clean and preprocess the data to prepare it for analysis. Handle missing values, normalize the data, and convert categorical data into numerical formats as needed.
3. Choose Machine Learning Models
Select appropriate machine learning models based on the problem you want to solve—be it classification, regression, or clustering. Models such as decision trees, random forests, and neural networks are commonly used in agricultural applications.
4. Train and Validate Your Model
Split your data into training and testing sets. Train your model on the training set and validate its performance on the unseen testing set to ensure it generalizes well.
5. Implement Insights into Practice
Once your model is validated, the next step is to apply the insights gained from the analysis to real-world farming practices. Monitor the impact of these changes to continuously improve your model.
The Future of Agriculture and Machine Learning
The integration of machine learning into agriculture is still in its early stages, but its potential is immense. As technology continues to evolve, we expect to see the following trends:
- Greater Adoption of AI Technologies: More farmers will adopt AI and machine learning technologies as they become more user-friendly and accessible.
- Improved Data Collection Methods: Advances in IoT devices and sensors will enhance data collection, providing more accurate and real-time data for analysis.
- Collaboration and Sharing of Data: Increased collaboration among farmers, researchers, and tech companies will lead to richer datasets and more comprehensive models.
- Focus on Sustainability: Machine learning will play a critical role in promoting sustainable farming practices, helping to address global food security and environmental concerns.
Conclusion
The potential of agriculture datasets for machine learning is revolutionizing the agriculture industry. By providing critical insights and fostering data-driven decision-making, these datasets help farmers improve yield, efficiency, and sustainability. As technology continues to advance, the convergence of agriculture and machine learning promises to yield even more remarkable results, shaping the future of farming for generations to come.
At Keymakr, we are committed to empowering businesses in the software development industry by harnessing the potential of data. Explore our various services and discover how we can help you thrive in the digital age.